Last update: August 14, 2024; AutoGen version: v0.2.35
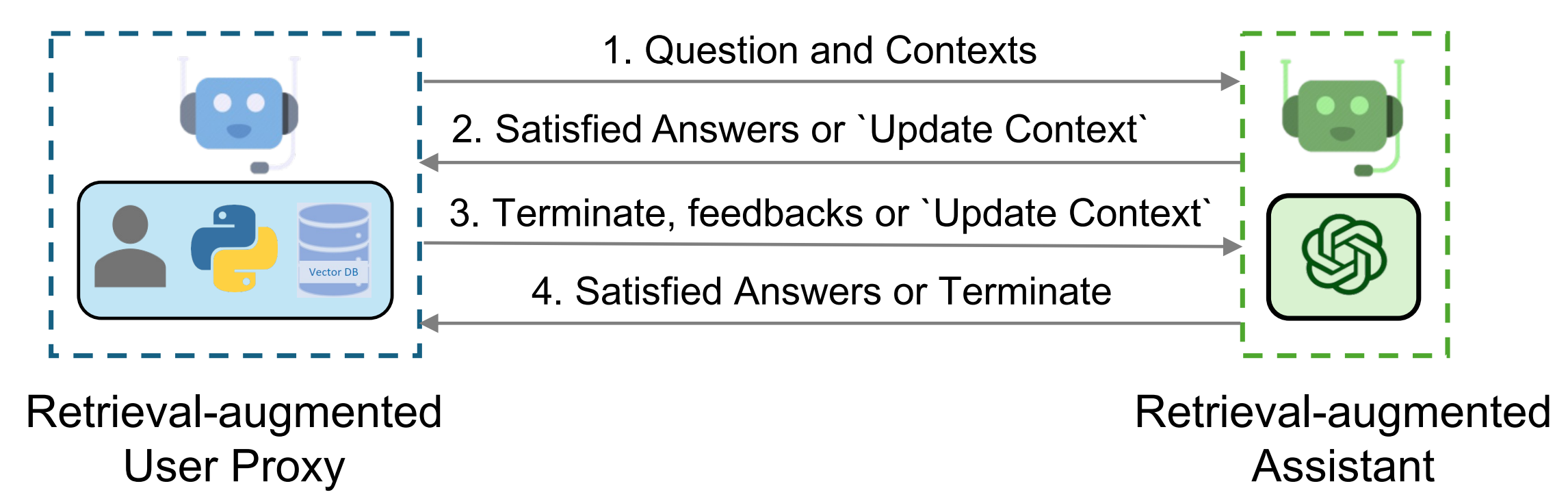
TL;DR:
- We introduce RetrieveUserProxyAgent, RAG agents of AutoGen that
allows retrieval-augmented generation, and its basic usage.
- We showcase customizations of RAG agents, such as customizing the embedding function, the text
split function and vector database.
- We also showcase two advanced usage of RAG agents, integrating with group chat and building a Chat
application with Gradio.
Introduction
Retrieval augmentation has emerged as a practical and effective approach for mitigating the intrinsic
limitations of LLMs by incorporating external documents. In this blog post, we introduce RAG agents of
AutoGen that allows retrieval-augmented generation. The system consists of two agents: a
Retrieval-augmented User Proxy agent, called RetrieveUserProxyAgent
, and an Assistant
agent, called RetrieveAssistantAgent
; RetrieveUserProxyAgent
is extended from built-in agents from AutoGen,
while RetrieveAssistantAgent
can be any conversable agent with LLM configured.
The overall architecture of the RAG agents is shown in the figure above.
To use Retrieval-augmented Chat, one needs to initialize two agents including Retrieval-augmented
User Proxy and Retrieval-augmented Assistant. Initializing the Retrieval-Augmented User Proxy
necessitates specifying a path to the document collection. Subsequently, the Retrieval-Augmented
User Proxy can download the documents, segment them into chunks of a specific size, compute
embeddings, and store them in a vector database. Once a chat is initiated, the agents collaboratively
engage in code generation or question-answering adhering to the procedures outlined below:
- The Retrieval-Augmented User Proxy retrieves document chunks based on the embedding similarity,
and sends them along with the question to the Retrieval-Augmented Assistant.
- The Retrieval-Augmented Assistant employs an LLM to generate code or text as answers based
on the question and context provided. If the LLM is unable to produce a satisfactory response, it
is instructed to reply with “Update Context” to the Retrieval-Augmented User Proxy.
- If a response includes code blocks, the Retrieval-Augmented User Proxy executes the code and
sends the output as feedback. If there are no code blocks or instructions to update the context, it
terminates the conversation. Otherwise, it updates the context and forwards the question along
with the new context to the Retrieval-Augmented Assistant. Note that if human input solicitation
is enabled, individuals can proactively send any feedback, including Update Context”, to the
Retrieval-Augmented Assistant.
- If the Retrieval-Augmented Assistant receives “Update Context”, it requests the next most similar
chunks of documents as new context from the Retrieval-Augmented User Proxy. Otherwise, it
generates new code or text based on the feedback and chat history. If the LLM fails to generate
an answer, it replies with “Update Context” again. This process can be repeated several times.
The conversation terminates if no more documents are available for the context.
Basic Usage of RAG Agents
- Install dependencies
Please install autogen with the [retrievechat] option before using RAG agents.
pip install "autogen[retrievechat]"
RetrieveChat can handle various types of documents. By default, it can process
plain text and PDF files, including formats such as 'txt', 'json', 'csv', 'tsv',
'md', 'html', 'htm', 'rtf', 'rst', 'jsonl', 'log', 'xml', 'yaml', 'yml' and 'pdf'.
If you install unstructured,
additional document types such as 'docx',
'doc', 'odt', 'pptx', 'ppt', 'xlsx', 'eml', 'msg', 'epub' will also be supported.
- Install
unstructured
in ubuntu
sudo apt-get update
sudo apt-get install -y tesseract-ocr poppler-utils
pip install unstructured[all-docs]
You can find a list of all supported document types by using autogen.retrieve_utils.TEXT_FORMATS
.
- Import Agents
import autogen
from autogen import AssistantAgent
from autogen.agentchat.contrib.retrieve_user_proxy_agent import RetrieveUserProxyAgent
- Create an 'AssistantAgent' instance named "assistant" and an 'RetrieveUserProxyAgent' instance named "ragproxyagent"
Refer to the doc
for more information on the detailed configurations.
assistant = AssistantAgent(
name="assistant",
system_message="You are a helpful assistant.",
llm_config=llm_config,
)
ragproxyagent = RetrieveUserProxyAgent(
name="ragproxyagent",
retrieve_config={
"task": "qa",
"docs_path": "https://raw.githubusercontent.com/microsoft/autogen/main/README.md",
},
)
- Initialize Chat and ask a question
assistant.reset()
ragproxyagent.initiate_chat(assistant, message=ragproxyagent.message_generator, problem="What is autogen?")
Output is like:
--------------------------------------------------------------------------------
assistant (to ragproxyagent):
AutoGen is a framework that enables the development of large language model (LLM) applications using multiple agents that can converse with each other to solve tasks. The agents are customizable, conversable, and allow human participation. They can operate in various modes that employ combinations of LLMs, human inputs, and tools.
--------------------------------------------------------------------------------
- Create a UserProxyAgent and ask the same question
assistant.reset()
userproxyagent = autogen.UserProxyAgent(name="userproxyagent")
userproxyagent.initiate_chat(assistant, message="What is autogen?")
Output is like:
--------------------------------------------------------------------------------
assistant (to userproxyagent):
In computer software, autogen is a tool that generates program code automatically, without the need for manual coding. It is commonly used in fields such as software engineering, game development, and web development to speed up the development process and reduce errors. Autogen tools typically use pre-programmed rules, templates, and data to create code for repetitive tasks, such as generating user interfaces, database schemas, and data models. Some popular autogen tools include Visual Studio's Code Generator and Unity's Asset Store.
--------------------------------------------------------------------------------
You can see that the output of UserProxyAgent
is not related to our autogen
since the latest info of
autogen
is not in ChatGPT's training data. The output of RetrieveUserProxyAgent
is correct as it can
perform retrieval-augmented generation based on the given documentation file.
Customizing RAG Agents
RetrieveUserProxyAgent
is customizable with retrieve_config
. There are several parameters to configure
based on different use cases. In this section, we'll show how to customize embedding function, text split
function and vector database.
Customizing Embedding Function
By default, Sentence Transformers and its pretrained models will be used to
compute embeddings. It's possible that you want to use OpenAI, Cohere, HuggingFace or other embedding functions.
from chromadb.utils import embedding_functions
openai_ef = embedding_functions.OpenAIEmbeddingFunction(
api_key="YOUR_API_KEY",
model_name="text-embedding-ada-002"
)
ragproxyagent = RetrieveUserProxyAgent(
name="ragproxyagent",
retrieve_config={
"task": "qa",
"docs_path": "https://raw.githubusercontent.com/microsoft/autogen/main/README.md",
"embedding_function": openai_ef,
},
)
huggingface_ef = embedding_functions.HuggingFaceEmbeddingFunction(
api_key="YOUR_API_KEY",
model_name="sentence-transformers/all-MiniLM-L6-v2"
)
More examples can be found here.
Customizing Text Split Function
Before we can store the documents into a vector database, we need to split the texts into chunks. Although
we have implemented a flexible text splitter in autogen, you may still want to use different text splitters.
There are also some existing text split tools which are good to reuse.
For example, you can use all the text splitters in langchain.
from langchain.text_splitter import RecursiveCharacterTextSplitter
recur_spliter = RecursiveCharacterTextSplitter(separators=["\n", "\r", "\t"])
ragproxyagent = RetrieveUserProxyAgent(
name="ragproxyagent",
retrieve_config={
"task": "qa",
"docs_path": "https://raw.githubusercontent.com/microsoft/autogen/main/README.md",
"custom_text_split_function": recur_spliter.split_text,
},
)
Customizing Vector Database
We are using chromadb as the default vector database, you can also use mongodb, pgvectordb and qdrantdb
by simply set vector_db
to mongodb
, pgvector
and qdrant
in retrieve_config
, respectively.
To plugin any other dbs, you can also extend class agentchat.contrib.vectordb.base
,
check out the code here.
Advanced Usage of RAG Agents
Integrate with other agents in a group chat
To use RetrieveUserProxyAgent
in a group chat is almost the same as you use it in a two agents chat. The only thing is that
you need to initialize the chat with RetrieveUserProxyAgent
. The RetrieveAssistantAgent
is not necessary in a group chat.
However, you may want to initialize the chat with another agent in some cases. To leverage the best of RetrieveUserProxyAgent
,
you'll need to call it from a function.
boss = autogen.UserProxyAgent(
name="Boss",
is_termination_msg=termination_msg,
human_input_mode="TERMINATE",
system_message="The boss who ask questions and give tasks.",
)
boss_aid = RetrieveUserProxyAgent(
name="Boss_Assistant",
is_termination_msg=termination_msg,
system_message="Assistant who has extra content retrieval power for solving difficult problems.",
human_input_mode="NEVER",
max_consecutive_auto_reply=3,
retrieve_config={
"task": "qa",
},
code_execution_config=False,
)
coder = autogen.AssistantAgent(
name="Senior_Python_Engineer",
is_termination_msg=termination_msg,
system_message="You are a senior python engineer. Reply `TERMINATE` in the end when everything is done.",
llm_config={"config_list": config_list, "timeout": 60, "temperature": 0},
)
pm = autogen.AssistantAgent(
name="Product_Manager",
is_termination_msg=termination_msg,
system_message="You are a product manager. Reply `TERMINATE` in the end when everything is done.",
llm_config={"config_list": config_list, "timeout": 60, "temperature": 0},
)
reviewer = autogen.AssistantAgent(
name="Code_Reviewer",
is_termination_msg=termination_msg,
system_message="You are a code reviewer. Reply `TERMINATE` in the end when everything is done.",
llm_config={"config_list": config_list, "timeout": 60, "temperature": 0},
)
def retrieve_content(
message: Annotated[
str,
"Refined message which keeps the original meaning and can be used to retrieve content for code generation and question answering.",
],
n_results: Annotated[int, "number of results"] = 3,
) -> str:
boss_aid.n_results = n_results
_context = {"problem": message, "n_results": n_results}
ret_msg = boss_aid.message_generator(boss_aid, None, _context)
return ret_msg or message
for caller in [pm, coder, reviewer]:
d_retrieve_content = caller.register_for_llm(
description="retrieve content for code generation and question answering.", api_style="function"
)(retrieve_content)
for executor in [boss, pm]:
executor.register_for_execution()(d_retrieve_content)
groupchat = autogen.GroupChat(
agents=[boss, pm, coder, reviewer],
messages=[],
max_round=12,
speaker_selection_method="round_robin",
allow_repeat_speaker=False,
)
llm_config = {"config_list": config_list, "timeout": 60, "temperature": 0}
manager = autogen.GroupChatManager(groupchat=groupchat, llm_config=llm_config)
boss.initiate_chat(
manager,
message="How to use spark for parallel training in FLAML? Give me sample code.",
)
Build a Chat application with Gradio
Now, let's wrap it up and make a Chat application with AutoGen and Gradio.
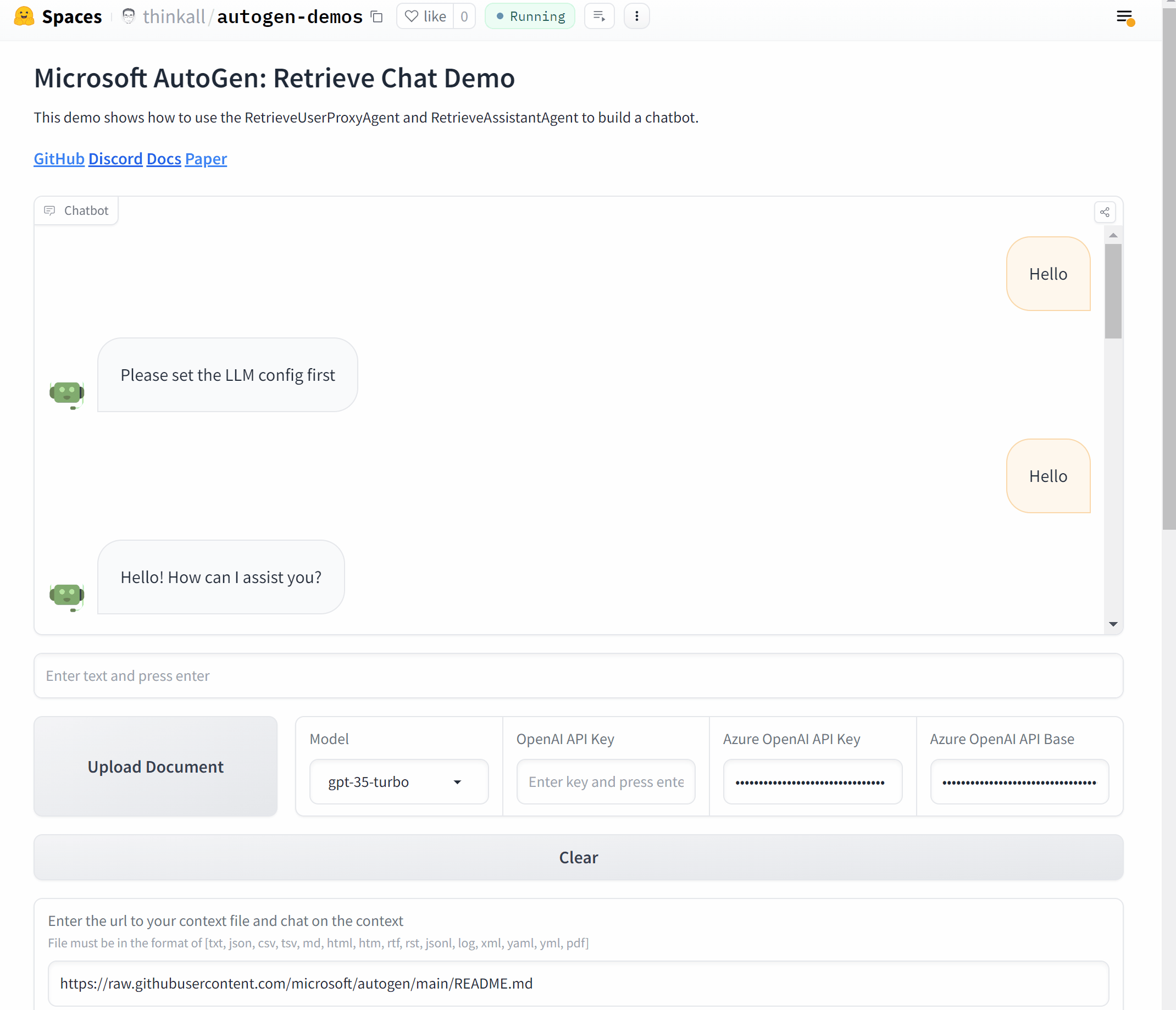
def initialize_agents(config_list, docs_path=None):
...
return assistant, ragproxyagent
def initiate_chat(config_list, problem, queue, n_results=3):
...
assistant.reset()
try:
ragproxyagent.a_initiate_chat(
assistant, problem=problem, silent=False, n_results=n_results
)
messages = ragproxyagent.chat_messages
messages = [messages[k] for k in messages.keys()][0]
messages = [m["content"] for m in messages if m["role"] == "user"]
print("messages: ", messages)
except Exception as e:
messages = [str(e)]
queue.put(messages)
def chatbot_reply(input_text):
"""Chat with the agent through terminal."""
queue = mp.Queue()
process = mp.Process(
target=initiate_chat,
args=(config_list, input_text, queue),
)
process.start()
try:
messages = queue.get(timeout=TIMEOUT)
except Exception as e:
messages = [str(e) if len(str(e)) > 0 else "Invalid Request to OpenAI, please check your API keys."]
finally:
try:
process.terminate()
except:
pass
return messages
...
with gr.Blocks() as demo:
...
assistant, ragproxyagent = initialize_agents(config_list)
chatbot = gr.Chatbot(
[],
elem_id="chatbot",
bubble_full_width=False,
avatar_images=(None, (os.path.join(os.path.dirname(__file__), "autogen.png"))),
)
txt_input = gr.Textbox(
scale=4,
show_label=False,
placeholder="Enter text and press enter",
container=False,
)
with gr.Row():
txt_model = gr.Dropdown(
label="Model",
choices=[
"gpt-4",
"gpt-35-turbo",
"gpt-3.5-turbo",
],
allow_custom_value=True,
value="gpt-35-turbo",
container=True,
)
txt_oai_key = gr.Textbox(
label="OpenAI API Key",
placeholder="Enter key and press enter",
max_lines=1,
show_label=True,
value=os.environ.get("OPENAI_API_KEY", ""),
container=True,
type="password",
)
...
clear = gr.ClearButton([txt_input, chatbot])
...
if __name__ == "__main__":
demo.launch(share=True)
The online app and the source code are hosted in HuggingFace. Feel free to give it a try!
Read More
You can check out more example notebooks for RAG use cases: